Note: this post assumes some familiarity with the Nordic RK (“Regulerkraft” or “regulating energy”) power market. For those without such a background and who are interested in learning more, we would direct you to [Regulerkraftmarkedet | Statnett](https://www.statnett.no/for-aktorer-i-kraftbransjen/systemansvaret/kraftmarkedet/reservemarkeder/tertiarreserver/regulerkraftmarkedet/) (in Norwegian)
Our experience in using ML for operational forecasting has shown us that no single ML forecasting model is great at forecasting all market behaviour and in all time periods. Why? Well, in developing and training such a model, you have to make trade-offs. A single model needs to perform well in all time periods - otherwise its unreliability would make it difficult to use consistently. More often than not, trying to develop a single model means you have to trade off accuracy in one aspect or situation with accuracy in another. This gives models that are just OK - never really poor, but never able to perform well.
Instead, our approach - and what we deliver in our fab:app forecasts - is the use of multiple targeted or specialised models, where each one is designed to focus on one aspect of the market in question.
So, for example, for our Nordic RK market forecasts, we have one ensemble model that forecasts the price distribution in the upcoming hours, and another set of models that focus on large price RK-spot price spread, among others.
To illustrate the benefits of this, we can examine the fab:rk nordic price forecasts for NO2 from the morning of May 13, 2022. At 6am, our quantile price model forecast rk prices to most likely be distributed around the day-ahead price level, with the median forecast being at or close to spot.
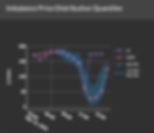
This is what occurred until 13:00, when the market became up-regulated with a price of 174 €/MWh. Such a price movement was not impossible according to the quantile forecast; however, the quantile forecast in and of itself provided little indication that this may occur.
So, what can we do about this? This is where our LPI - Large Price Index - models come in to play. These models focus solely on indicating the likelihood of large rk price spreads to spot. An index value of 0 indicates very low likelihood, whilst a value of 10 indicates high likelihood.
We provide 2 LPIs, each produced by its own specialised models - a base, and a conditional. The base LPI gives an indication of the overall likelihood that the market will be up (or down) regulated AND that the rk-spot spread will be large; the conditional gives the likelihood of a large rk-spot spread _if_ the market is up (or down) regulated.
The “Up” base LPI answers the question:
What is the likelihood that the market is up-regulated AND rk-spot spread is high?
The conditional “Up” LPI answers the question:
If the market ends up being up-regulated, what is the chance that the rk-spot spread is high?
Let’s have a look at both LPIs also produced at 6am on May 13th. Firstly, the base up LPI:
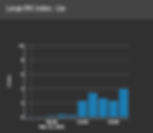
And secondly the conditional up LPI:
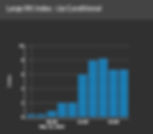
The base up LPI forecast very low chances of up-regulation and large deviations in the morning hours, followed by an increased but still moderate likelihood from 12 noon. Similarly, the conditional up LPI forecast low likelihood of large rk-spot spreads in the morning hours even if the market were up-regulated. From 12 noon however, the likelihood was that, if the market were up-regulated, the rk-spot spread would be substantial. This is indeed what occurred.
This illustrates very clearly the advantages of multiple, specialised models over a single forecast. Alone, the quantile model provided little distinction between the morning hours and those in the afternoon. Adding the LPI forecasts provided a strong separation between the two time periods, and indicated a substantial risk in the afternoon of high RK prices should the market require up regulation.
The provision of this type of additional subtleties in a forecast is essential if the forecast is to be consistently valuable. By using specialised models designed for specific and different forecasting tasks, we can gain benefits and insights that single models are unable to provide. And by designing models specifically for these tasks, we ensure they are as good as they can be at them.